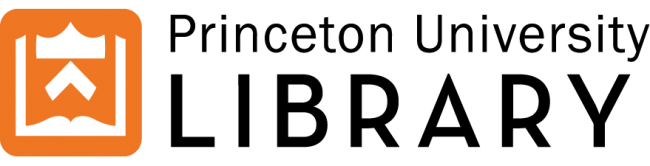
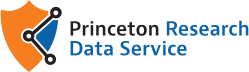

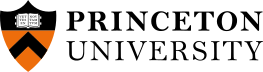
In the attention schema theory, the brain constructs a model of attention, the attention schema, to aid in the endogenous control of attention. Growing behavioral evidence appears to support this proposal. However, a central question remains: does a controller of attention actually benefit by having access to an attention schema? We constructed an artificial, deep Q-learning, neural network agent that was trained to control a simple form of visuospatial attention, tracking a stimulus with its attention spotlight in order to solve a catch task. The agent was tested with and without access to an attention schema. In both conditions, the agent received sufficient information such that it should, theoretically, be able to learn the task. We found that with an attention schema present, the agent learned to control its attention spotlight and learned the catch task to a high degree of performance. Once the agent learned, if the attention schema was disabled, the agent could no longer perform effectively. If the attention schema was removed before learning began, the agent was drastically impaired at learning. The results show how the presence of even a simple attention schema provides a profound benefit to a controller of attention. We interpret these results as supporting the central argument of AST: the brain evolved an attention schema because of its practical benefit in the endogenous control of attention. Download the README.txt file for a detailed description of this dataset's content This dataset contains csv files containing the results of three experiments described in the related manuscript as well as the python scripts used to build the model and run the experiments.
Show MoreFilename | Size |
---|