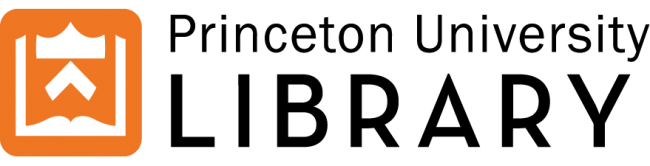
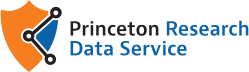

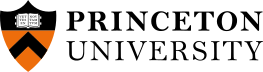
A dataset of 2400 quantum cascade structures at 15 electric field iterations, for a total of 36000 unique designs. The structures are generated by randomly altering a starting 10-layer design of alternating Al0.48In0.52As barrier material and In0.53Ga0.47As well material, with layer thickness sequence of 9/57/11/54/12/45/25/34/14/33 Angstroms (starting with well material). The random tolerance range is from -5 to +20 Angstroms in 5 Angstrom increments. The laser transition Figure of Merit, among other quantities of interest, is identified for each design using a method found in: A. C. Hernandez, M. Lyu and C. F. Gmachl, "Generating Quantum Cascade Laser Datasets for Applications in Machine Learning," 2022 IEEE Photonics Society Summer Topicals Meeting Series (SUM), 2022, pp. 1-2, doi: 10.1109/SUM53465.2022.9858281
Show MoreFilename | Size |
---|